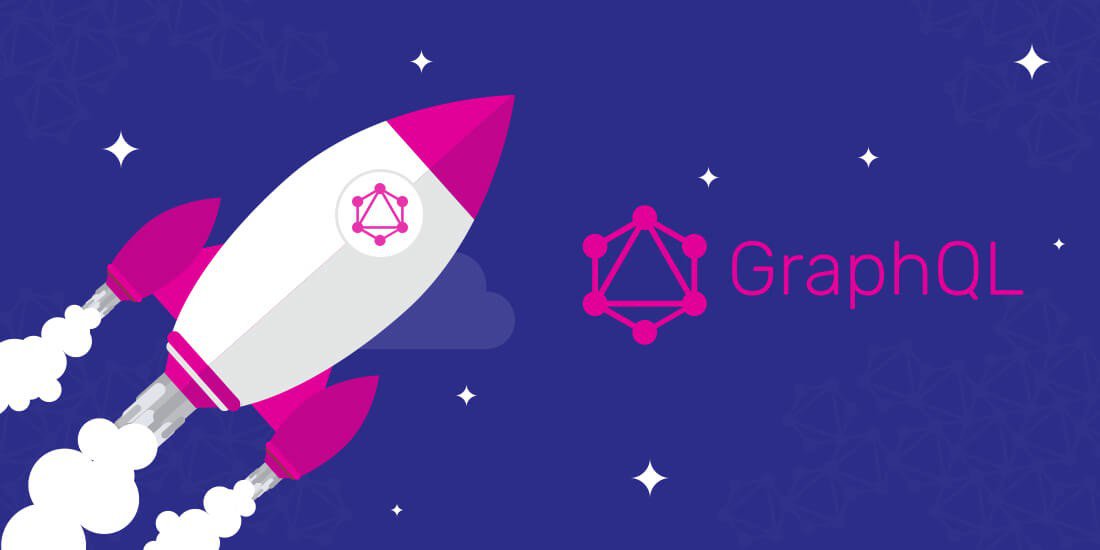
Modern apps are chatty—they require a lot of data, and thus make a lot of requests to underlying services to fulfill those needs. Layering GraphQL over your services solves this issue, since it encapsulates multiple requests into a single operation, avoiding the cost of multiple round trips.
Apollo makes it easy to compose your application from individual components that manage their own data dependencies. This pattern enables you to grow your app and add new features without risk of breaking existing functionality. It does however come with a performance cost as each component will fire off uncoordinated GraphQL queries as they are being mounted by React.
In this article we will dive into transport-level query batching, an advanced Apollo feature that enables you to significantly improve performance of complex applications.
By the end of this post, you should be able to answer the following questions about batching client operations with Apollo:
- How does batching work?
- What are the tradeoffs with batching?
- Is batching necessary?
- Can batching be done manually?
- Can I fix automatic batching?.
. . .
How does batching work?
Batching is the process of taking a group of requests, combining them into one, and making a single request with the same data that all of the other queries would have made. This is usually done with a timing threshold.
In GraphQL apps, batching usually takes one of two forms. The first form takes all operations and combines them into a single operation using the alias feature in GraphQL. This approach is not recommended, however, since this removes the ease of tracking metrics on a per-operation basis and adds additional complexity to the client.
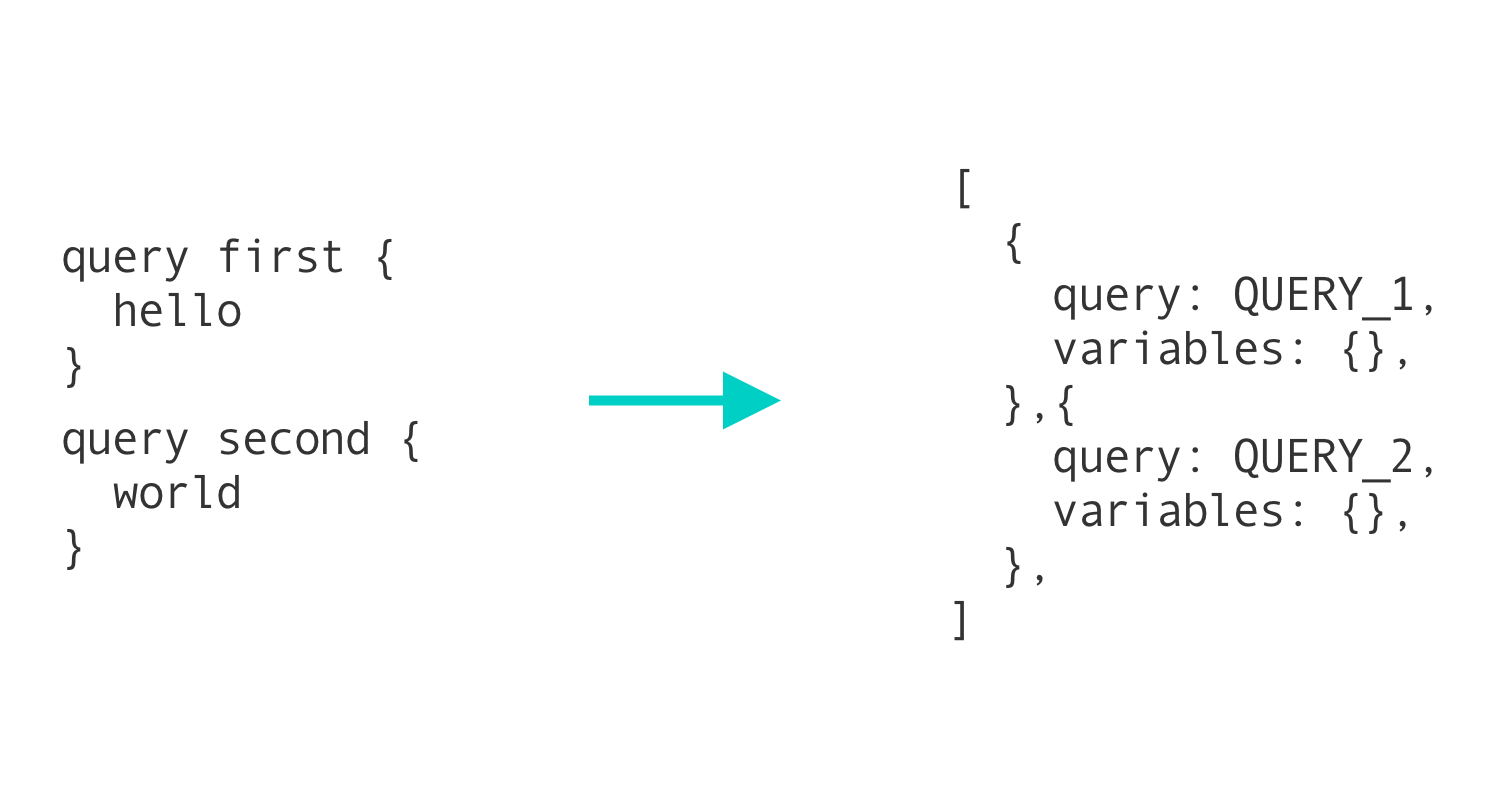
For a deeper dive into how batching works with Apollo, check out this post introducing batching in an earlier version of Apollo Client. Even though some of the implementation details have changed, the concepts are still relevant today.
. . .
The Example App
We will use an extended version of the Learn Apollo Pokedex app to explore the performance gains query batching can provide. The original Pokedex app lists all Pokemon for a single trainer. We make the app multi-tenant by rendering the Pokedex component for each trainer. This is how the app looks like with 6 trainers.
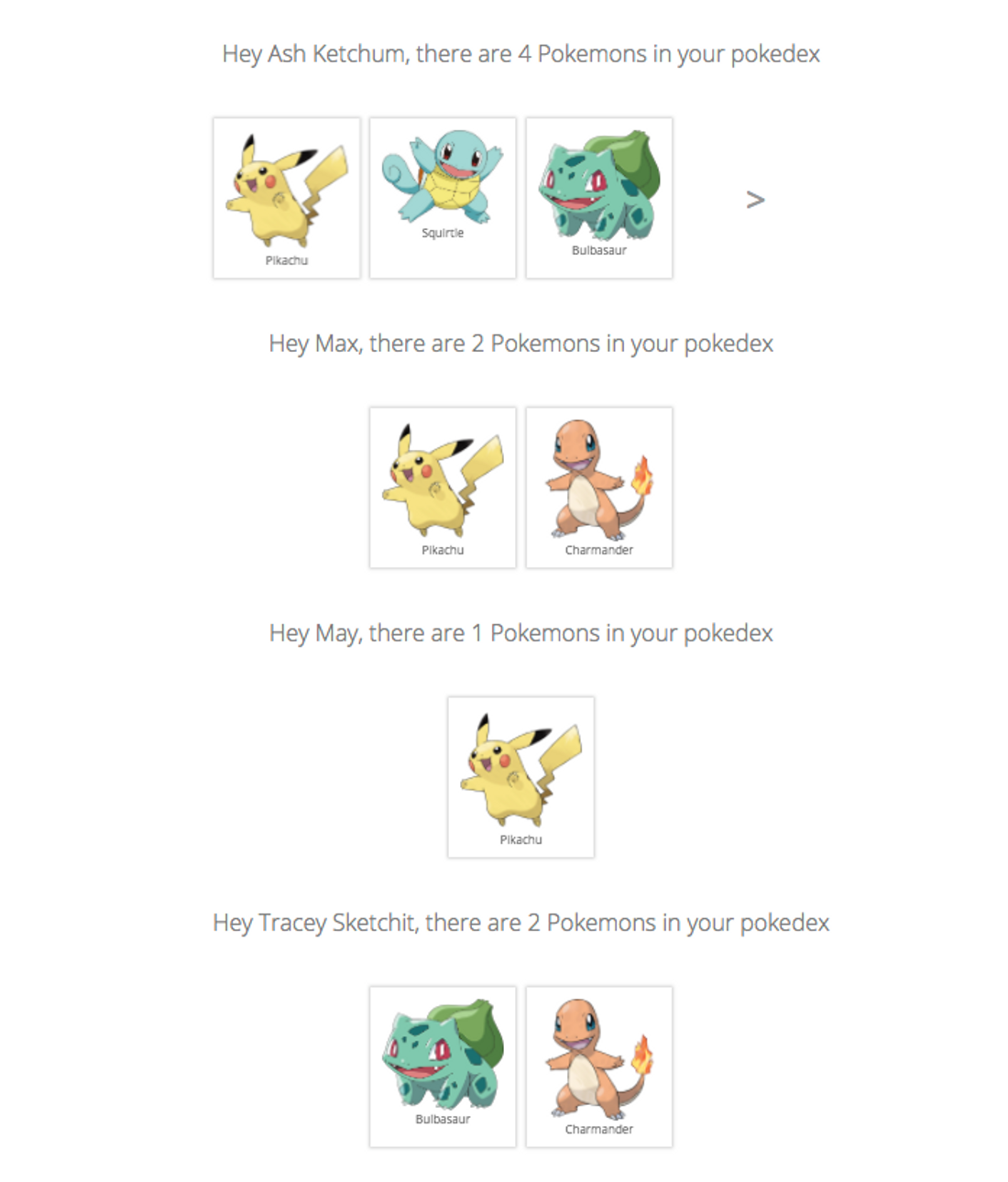
1. Before Batching Queries
Chrome DevTools has a very detailed network traffic inspection feature. If you are serious about app performance take a look at the documentation. When you load the extended pokedex and filter for requests to the GraphQL backend it looks like this
The first thing you notice is that Apollo is generating 12 requests. This makes sense as we are rendering 12 Pokedex components. Each request takes around 100 ms and the first 6 requests completes within 126 ms. But now something interesting happens.
The following 6 requests are stalled for up to 126 ms while the first requests complete. All browsers have a limit on concurrent connections. For Chrome the limit is currently 6 concurrent requests to the same hostname, so 7 requests will take roughly double the amount of time to complete as 6 requests.
2. After Batching Queries
This is where Apollos Query Batching comes into play. If Query Batching is enabled, Apollo will not issue requests immediately. Instead it will wait for up to 10 ms to see if more requests come in from other components. After the 10 ms, Apollo will issue 1 request containing all the queries. This eliminates the issue with stalled connections and delivers significantly better performance
The performance of this combined query is almost as good as a single query from the first test.
. . .
Manually Batching Queries
Batching can be done manually. Often, for the cases where batching may still be a necessity, inexpensive operations can be manually batched together to prevent unnecessary requests. In GraphQL, this is done by combining smaller queries into one larger one.
For example, if there was a page with four content blocks on it, rather than having each block fetch its own data, a container could fetch the data and pass it to the components manually. This is conceptually similar to the first implementation of batching described in the first section.
This may sound counterintuitive to the patterns that have been established, like colocating queries with the components that use their response, but there are ways around this.
This isn’t suggesting to write one large GraphQL query at the container-level. Instead, write queries normally, next to the components that use them. When you’re ready to optimize a section of an app, convert those queries to fragments and export them from the component file.
You can then import these fragments in the container, let the container make the single, large query, and pass the fragment results back to the children. Using container components in this way can even allow you to control loading and error states at the container-level, rather than in each component.
Take a look at this CodeSandbox for a full example of this in action:
Even manual batching has issues, though. Since manually batched operations are much larger,their ability to take advantage of whole-query caching is reduced. Whole-query cache TTLs are based on the field in an operation with the shortest TTL.
Increasing the number of fields in an operation increases the chances that a field that can’t be cached for a long time is included, reducing the ability to cache the whole operation. For more on whole-query caching, and how these TTLs are calculated, read this doc.
. . .
Automatic batching (Apollo Client)
There is no silver bullet for batching. If batching is enabled, there is always the potential for portions of the batch to run slower, and thus hold up the remaining portions of the batch. Sometimes, however, the trouble of manually batching operations outweighs the benefits. Manually batching may be too complicated, or too large of a refactor to reasonably undertake.
Enabling batching in Apollo Client:
import ApolloClient, { createBatchingNetworkInterface } from *'apollo-client'*
const client = new ApolloClient({
networkInterface: createBatchingNetworkInterface({ uri: *'https://api.graph.cool/simple/v1/ciybssqs700el0132puboqa9b', * batchInterval: 10}),
dataIdFromObject: o => o.id
})
Some of the issues around batching can be solved by manually debatching expensive operations–that is, allowing most operations to be batched like normal, but preventing batching for ones that are known to cause issues. Doing this requires a few steps:
- Build components as usual, with colocated queries.
- Identify the most expensive operations using a tool like Apollo Engine(these are not necessarily just the largest queries).
- Mark expensive operations on the client using an operation’s context. You can set the context by specifying a prop on your Query component.
- Use split to switch between apollo-link-http or apollo-link-batch-http depending on the context of the operation.
Take a look at this CodeSandbox for a full example of this in action:
. . .
Conclusion
Batching is a tricky topic. There are plenty of reasons to use some form of client request batching, but many times these solutions just cause more problems than they solve.
Hopefully, armed with this information, you can feel confident when making a decision about how to boost the performance of the client.
No comments:
Post a Comment